The power of artificial intelligence has transformed health care by using massive datasets to improve diagnostics, treatment, records management, and patient outcomes. Complex decisions that once took hours — such as making a breast or lung cancer diagnosis based on imaging studies, or deciding when patients should be discharged — are now resolved within seconds by machine learning and deep learning applications.
Any technology, of course, will have its limitations and flaws. And over the past few years, a steady stream of evidence has demonstrated that some of these AI-powered medical technologies are replicating racial bias and exacerbating historic health care inequities. Now, amid the SARS-CoV-2 pandemic, some researchers are asking whether these new technologies might be contributing to the disproportionately high rates of virus-related illness and death among African Americans. African Americans aged 35 to 44 experience Covid-19 mortality rates that are nine times higher than their White counterparts. Many African Americans also say they have limited access to Covid-19 testing.
During the early weeks of the pandemic, there were few — if any — Covid-19 testing locations in African American communities. Public health officials in states such as California, Illinois, Tennessee, and Texas have said that decisions about whom and where to test were data-driven and reflected the demographics of early cases. Yet the initial focus on affluent White communities allowed thousands of infections to quickly spread across cities and towns whose residents experience disproportionately high rates of underlying health conditions.
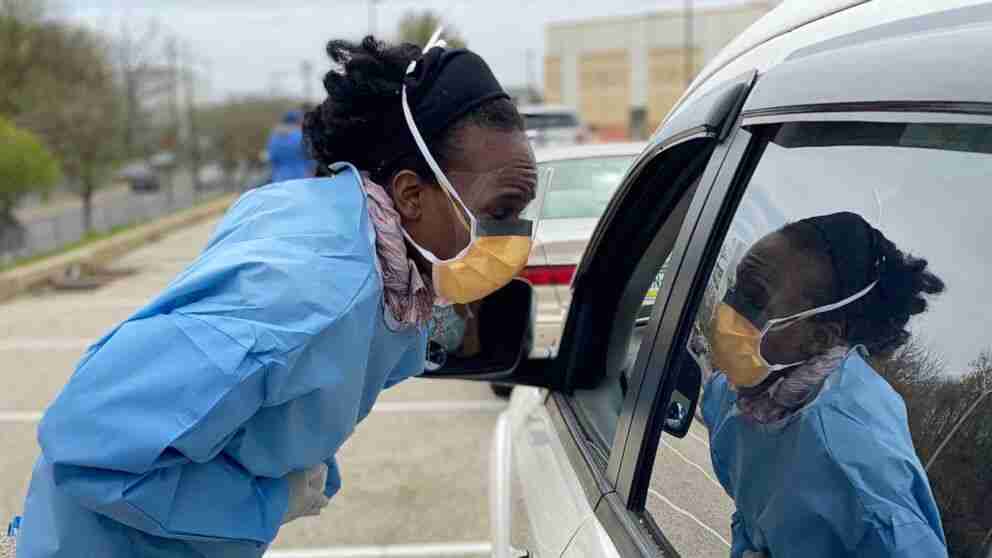
African American communities should have been prioritized for testing locations, says Alondra Nelson, a sociologist and president of the Social Science Research Council, an almost 100-year-old independent research organization that advances better understanding of social science. When algorithms overlooked health disparities as a risk factor, Nelson says, public health officials issued an implicit statement to African American communities: “We’re not going to triage and treat you.”
Those who study the problem say that the extent of racial bias in AI medical technologies is unknown. This is, in part, due to a lack of transparency: The software is often proprietary, which means the intellectual property — the process, inputs, and source coding — is protected and copyrighted. Independent researchers have very little, if any, access to the data. “We’re in a new space,” says Nelson. In many instances, big data is now a business product. This is why she and others say that fixing racial bias in AI may involve addressing not just science, but also policy and law.
The stakes are high. Algorithms affect the lives of virtually all Americans, making rapid, automated decisions in credit, finance, employment, compensation, housing, courts and sentencing, health, medicine, and other areas. These decisions are critical to our daily lives, and they are largely automated.
Last fall, a research team published a paper in the journal Science that for the first time attempted to quantify the extent of racial bias in patient care and outcomes. The researchers studied an algorithm developed by Optum, a subsidiary of the world’s largest health care company, UnitedHealth Group. Other companies, such as 3M Health Information Systems and Verisk Health, produce similar algorithms, as do some universities and the Centers for Medicare and Medicaid Services.
The algorithm under study was used to identify patients with complex medical needs and assign each what is known as a “risk score.” Patients with the highest scores are eligible for additional resources, such as home visits by a nurse, expedited primary care visits, and automatic prescription refills. The research team looked at how the algorithm performed at an unnamed medical center, which provided the health care records for nearly 50,000 patients. Almost 44,000 were White and about 6,000 were Black. Researchers compared the patients’ risk scores with their actual health data, including illness history and test results.
The way the medical center used the algorithm, the researchers concluded, was not neutral and unbiased. African Americans, for example, comprised just 18 percent of the medical center’s high-risk group when assessed using the algorithm. But when looking at actual patient health data, the Science researchers concluded the high-risk group should have been 47 percent African American. The discrepancy meant that White patients were granted access to resources ahead of African American patients who were less healthy.
The reason: The algorithm was trained to identify patients with higher anticipated future health care costs. It may seem reasonable to equate higher anticipated costs with deteriorating health, says the study’s lead author, Ziad Obermeyer, an emergency medicine physician and an associate professor at the University of California, Berkeley School of Public Health. But the formula privileges patients with higher incomes and top tier health insurance plans that cover preventive care, more doctor visits, higher cost prescriptions and such. It also ignores the reality that many low-income patients — who are disproportionately African American — are more likely to seek medical care only when their symptoms are severe.
The result: Poorer Black patients appear healthier than they actually are.
What’s “so striking” and “sadly very familiar” are that these algorithms are mimicking the structural racism evident across American society and in health care, says Obermeyer. These types of screening algorithms should “identify people who have the same health needs irrespective of the color of their skin,” Obermeyer adds. “That turns out not to be the case.”
In an email to Undark, Optum spokesperson Tyler Mason took issue with the Science analysis. “The study in question grossly mischaracterized a cost-prediction algorithm . . . based on one health system’s incorrect use of it, which was completely inconsistent with any recommended use of the tool.” That recommended use, according to Optum, is to model potential future health care costs for individual patients based on past health care experiences. It is not intended to assist doctors in making care decisions. The algorithm “does not result in racial bias when used for that purpose,” Mason added.
Obermeyer agreed that the algorithm is not racially biased when used as a predictor of cost. “It predicts cost equally well for Black and White patients,” he wrote in an email. But he disagreed with the implication that just one hospital was misusing the algorithm. “On the one hand, everyone knew it was a cost predictor; on the other hand, everyone was very obviously using it to predict risk,” he wrote. As Obermeyer sees it, the problem was systemic: Software developers, hospitals, insurers, researchers — “we were all just thinking about this . . . in a way that was subtly but importantly wrong.”
Because risk-prediction algorithms are widely used, some researchers are now asking if their use contributed to the significant racial disparities seen in Covid-19 treatment and access to testing.
The narrative around Covid-19 and African Americans has largely been about comorbidities, says Nelson. As a group, African Americans experience higher rates of many serious health problems, including hypertension and diabetes. These conditions make people more vulnerable to Covid-19. “If we know that African Americans are more likely to have poor health outcomes because they have these comorbidities,” says Nelson, then they, as well as Latinos and Indigenous people, should have been prioritized for testing.
But that doesn’t appear to have happened. Nelson points to “lots of stories of people who have gone to the hospitals and emergency rooms two, three, four times,” and still testing was denied because “it was not believed that they were sick enough. These were African Americans who had Covid-19” and some later died, she adds.
Obermeyer recently spent time working at a hospital in Navajo Nation, a Native American reservation with some of the highest per capita Covid-19 infection rates in the United States. Navajo Nation’s health care infrastructure is “under-resourced,” says Obermeyer, and that can create a vicious cycle, similar to the one his team uncovered in their study of the AI-powered algorithm: If an underserved population lacks access to Covid-19 testing, then that population might look like it’s doing relatively well, even when it isn’t. This, in turn, may lead to fewer resources being allocated — and a delayed understanding of the full scope of the problem.
Racial bias has also been demonstrated in AI-powered medical diagnostic applications with a very particular nuance: The algorithms are less accurate diagnosing conditions on darker skin.
The phenomenon is not new. Facial recognition systems used by law enforcement, for example, are notoriously less accurate at identifying African American faces. Some of the relatively few studies documenting the problem have suggested up to 10 percent less accuracy compared to White faces. Self-driving auto technologies are also less accurate at recognizing pedestrians with darker skin — a situation that could have fatal consequences.
In medicine, machine learning has been used to create programs capable of distinguishing between images of benign and malignant moles. But a 2018 paper in JAMA Dermatology warned that “no matter how advanced the [machine learning] algorithm, it may underperform on images of lesions in skin of color.” This is because the training sets for the algorithms are not diverse, says Adewole Adamson, a dermatologist and assistant professor at the University of Texas at Austin Dell Medical School.
For the JAMA Dermatology paper, Adamson and his co-author studied sample data from the International Skin Imaging Collaboration: Melanoma Project, an open source dataset of more than 20,000 images of skin lesions. Collected from Australia, Europe, and the United States, the overwhelming majority of these images are from people with lighter complexions, according to Adamson.
Melanomas are relatively rare among African Americans. But they are often diagnosed at later stages — as is the case with most cancers — and their melanoma mortality rates are relatively high compared to Whites. The five-year survival rate among Whites for melanoma is 94 percent, according to the American Cancer Society. The rate is only 66 percent among Blacks.
Clinically-validated AI-powered apps that diagnose melanoma are not yet available to the average physician, but that may change soon. Numerous programmers are trying to develop and market the technology. “If you’re going to create such a program, you’re going to have to make sure that the skin images represented are representative of the different skin types that exist in the world,” said Adamson, who has conducted extensive research on racial disparities in dermatology.
Absent that, “you’re going to have to have some type of black box warning,” he continues: “Training sets are very important and it is critical in having a training set that represents what reality is.”
Some researchers are developing new mobile health applications with these realities in mind. For example, a team based at the University of California, San Francisco is developing a smartphone app that screens for diabetes. Users turn on the phone’s flashlight, place their fingertip over the phone’s camera lens, and then an optical technique known as photoplethysmography (PPG) allows the algorithm to extract features such as blood pressure and heart rate. The team is developing the application with Azumio, a mobile health technology company.
The algorithm was originally developed with data from about 54,000 participants enrolled in an online heart study. The participants were familiar with using smartphones to monitor their health, but they were less racially diverse than the broader U.S. population, says Robert Avram, a cardiologist and adjunct instructor at the University of California, San Francisco, and the lead author of a developing paper on this application. To ensure the algorithm is just as effective on darker complexions, the researchers are conducting extra rounds of clinical trials on African Americans and Asian Americans. Both groups have higher rates of diabetes than the general population.
Researchers across disciplines agree that broadening training sets is crucial to developing medical algorithms that perform equally well for all patients. “If Blacks, or Hispanics or other underrepresented groups are not included as a part of that training set, there may be unique features that are not going to be recognized by those algorithms,” says Renã A.S. Robinson, associate professor of chemistry at Vanderbilt University who is researching, among other things, a possible molecular basis for some racial disparities in Alzheimer’s disease. If those unique features go unnoticed, she adds, that could hinder physicians’ ability to detect disease early and provide the best treatment.
Researchers believe almost one-third of the world’s stored data is health care related. New “training data” is created each second, in the form of updated prescriptions, imaging reports, insurance bills, and more. All of this gets sorted and added to the archive created by various software developers. Developers generally block third parties — such as researchers — from accessing their programming code.
“There are a lot of frictions that prevent researchers from the outside from studying these things in a hospital or a health system where the data inevitably lives,” says Obermeyer. In his case, he was fortunate to be at a health system that purchased Optum’s algorithm. The health system allowed Obermeyer to use the software and access the data without cost for research purposes. The institutional review board approved the research.
For businesses, sharing a proprietary algorithm with an outside researcher also raises “the real issue of privacy and civil liberties,” says Nelson. It’s important for the company not to release personally identifiable data.
After the publication of the paper in Science, two agencies in the New York state government — the Departments of Financial Services and Health — announced investigations into UnitedHealth Group. Currently, there is no regulation or public oversight of algorithms, but a number of policy proposals have emerged in recent months.
Some politicians want federal agencies and health care companies to provide information on how they are responding to racial bias in algorithms. Sens. Cory Booker and Ron Wyden, along with U.S. Rep. Yvette Clarke, have co-sponsored the Algorithmic Accountability Act. The bill would compel certain companies to investigate many of their AI applications for bias. The bill would generally target larger companies, such as those with revenue in excess of $50 million per year, or those that collect personal data on 1 million or more consumers. The bill would also apply to data brokers such as Experian.
There have also been policy recommendations in recent months toward reducing algorithmic bias in health and medical applications. One of the more innovative suggestions: modernizing the Civil Rights Act of 1964 and making the case that it already applies to decisions made by artificial intelligence. The proposal was made in congressional testimony by Nicol Turner Lee, a sociologist and director of the Center for Technology Innovation at the Brookings Institution who conducts research at the intersections of race, social justice, and technology.
“The tech companies were operating in the ‘wild, wild west’ without any guard rails,” Turner Lee tells Undark. “So without risking the types of innovations that we’re seeing, it was important to [find] settled laws that define what you can and cannot do to a protected group or class.” She also adds: “I’ve been saying to legislators … that it’s very important to make a statement that the civil rights laws that have already been previously litigated apply to AI. Don’t let there be a gap.”
All of the sources interviewed for this article believe that the private sector has a critical role in innovation and developing technologies. But they all agreed that changes have to be made to reduce racial bias.
Obermeyer and his team take a slightly different approach from Turner Lee. Obermeyer believes that, at least for now, many health and medical stakeholders can develop best practices to reduce racial bias. Obermeyer says his team has been approached by health care systems, insurers, software developers, as well as state and federal regulators.
The researchers have offered to audit their algorithms on a pro-bono basis, and deliver recommendations on how to mitigate any biases that are discovered. This likely is the first time that stakeholders’ AI have been audited for fairness. Hopefully, says Obermeyer, this can “begin a conversation” to “share methods and best practices.”
Rod McCullom is a science journalist in Chicago. His work has been published by Undark, Scientific American, Nature, The Atlantic, and The Nation, among other publications. Find Rod on Twitter @rodmccullom
A version of this article was originally published at Undark and has been republished here with permission. Undark can be found on Twitter @undarkmag